Study Notes
What is derived demand?
- Level:
- AS, A-Level, IB
- Board:
- AQA, Edexcel, OCR, IB, Eduqas, WJEC
Last updated 1 Oct 2024
Derived demand refers to the demand for a particular product or resource that is driven by the demand for another product or resource. In other words, the demand for one item is derived from the demand for another item that is connected in the production or distribution process.
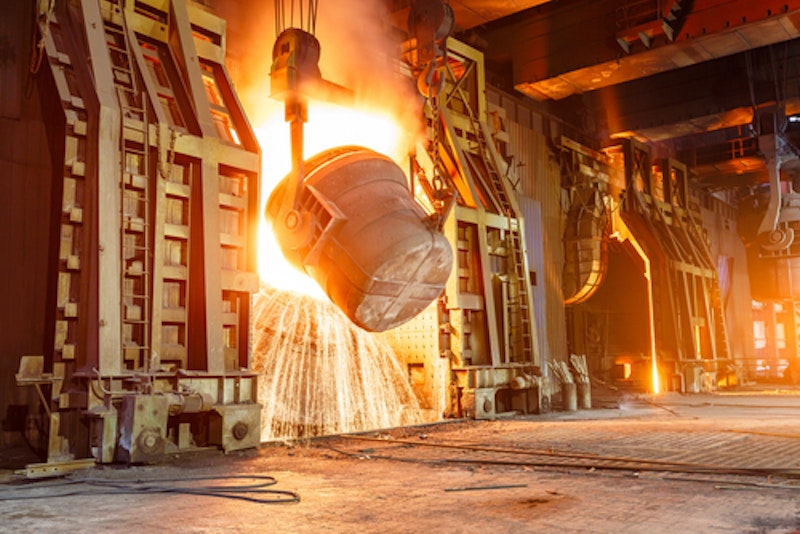
Derived demand occurs when the demand for a product or service is dependent on the demand for another product or service that is further down the supply chain.
It is typically observed in business-to-business (B2B) relationships and industrial markets.
For example, consider the demand for steel. Steel is not directly consumed by end consumers but is rather used as an input in various industries, such as construction, automotive manufacturing, and machinery production. The demand for steel is derived from the demand for these industries' final products. If the demand for automobiles increases, it will lead to an increase in the demand for steel to produce the necessary components.
Another example is the demand for labour. The demand for labour is derived from the demand for goods and services that require human resources for production. If a company experiences higher demand for its products, it may need to hire more workers to meet that demand.
In summary, derived demand refers to the indirect demand for a product or resource, which is driven by the demand for other related products or resources further down the supply chain. It highlights the interconnectedness of different stages of production and the interdependence of industries in the economy.
Derived demand can also be used to explain why the prices of some goods and services are so volatile. For example, the price of oil is often volatile because it is a derived demand. The demand for oil is derived from the demand for gasoline, diesel fuel, and other petroleum products. If the demand for these products increases, the demand for oil will also increase. This can lead to a sharp increase in the price of oil.
Generative AI and the Derived Demand for Power
Generative AI refers to artificial intelligence systems that can create new content, such as text, images, audio, or even code, based on the data they've been trained on. These models, such as OpenAI’s GPT models, are designed to generate human-like content by learning from vast amounts of information. They are widely used in applications like chatbots, content creation, image generation, and even in areas like scientific research or creative design.
Key Features of Generative AI:
- Content Creation: Generative AI can produce new content, such as writing articles, generating images, composing music, or designing objects, which traditionally required human input.
- Large-Scale Models: These AI systems are built on deep learning techniques, often using neural networks with millions or even billions of parameters, requiring large datasets and significant computational power to train and run.
- Adaptability: Generative AI models can adapt to various use cases, generating different forms of content depending on the prompt or input given.
Derived Demand for Power:
The concept of derived demand refers to demand for a factor of production that arises due to the demand for another product or service. In this case, the increasing use of generative AI has led to a derived demand for power (electricity), especially computational power, for several reasons:
- Training Large AI Models:
- Training large generative AI models requires significant computing power, especially in data centers using high-performance hardware like GPUs (Graphics Processing Units) or TPUs (Tensor Processing Units). These models are trained using huge datasets, often over several weeks or months, consuming vast amounts of energy.
- Example: Training a large language model like GPT requires enormous computational power due to the sheer volume of data and the complexity of the computations. This leads to a substantial demand for electricity to power the servers and cooling systems in data centers.
- Running and Inference:
- Once a model is trained, it still requires significant computing resources to operate, especially in real-time applications like chatbots or image generation. Every time someone interacts with an AI model, the system runs inference tasks to generate a response or content, which consumes power.
- Example: Running a generative AI service that processes millions of user queries or generates millions of images per day creates a high demand for servers, which, in turn, consume a lot of energy to perform these tasks continuously.
- Cloud Computing and Data Centers:
- Generative AI models are often hosted on cloud platforms (e.g., AWS, Google Cloud, Microsoft Azure), which rely on massive data centers. These data centers consume large amounts of electricity for both running AI models and for cooling systems to maintain the servers’ performance and prevent overheating.
- Example: Data centers hosting AI services are major consumers of electricity, often using energy-intensive cooling technologies to dissipate the heat generated by the servers processing AI tasks.
- AI at the Edge:
- There is also growing interest in running AI applications on edge devices, like smartphones or IoT devices, where power constraints become a crucial consideration. While less energy-intensive than large-scale data centers, these applications still drive demand for optimized hardware that can perform AI tasks efficiently with limited power consumption.
- Example: Running generative AI models on devices like smartphones for tasks such as real-time language translation or image enhancement increases the power demand in those devices, impacting overall energy consumption in consumer electronics.
Environmental and Economic Considerations:
- Energy Consumption and Sustainability: The vast amounts of power consumed by generative AI systems raise concerns about the environmental impact, particularly in terms of carbon emissions. As AI models grow larger and more prevalent, the demand for renewable energy sources and efficient data center infrastructure becomes more critical.
- Economic Impact: The derived demand for power affects energy markets, as more electricity is required to meet the needs of AI companies and data centers. This can influence energy prices and spur investment in energy-efficient technologies or alternative energy sources (like solar or wind power) to supply data centers sustainably.
Conclusion:
The rise of generative AI has created a significant derived demand for power, driven by the computational intensity of training and running large AI models. This demand impacts the energy sector, leading to increased pressure on power supply and infrastructure, and creates economic and environmental challenges as AI adoption continues to expand. Efficient power usage and sustainable energy solutions are becoming key considerations in the further development of AI technologies.
You might also like
Determinants of Demand (Revision Presentation)
Teaching PowerPoints
Market Mechanism Revision Quiz
Quizzes & Activities
Different Types of Demand
Topic Videos
Markets in Action - Crude Oil Prices
Topic Videos
IB Economics - The Law of Demand
Study Notes